Based Jensen Huang, CEO of Nvidia, which is one of the largest companies in the world by market cap now, said this February that people should stop learning to code. He was making the point that people will soon be able to instruct computers with natural human language to get what they want out of them. If you’re a younger person or a parent, it’s worth asking now, is there still value in learning to program a computer or get a computer science education?
I respect Jensen enormously and believe he is worth taking seriously, even if he used to slang 3D-Sexy-Elf boxes to gamers. Also to recognize his bias as the manufacturer of the picks and shovels for the current venture gold rush and his incentives to pump up the future perceived value of his company’s stock, if such a thing is even possible at a P/E of 73.
My perspective is as a software practitioner, having been a professional software “engineer” for two decades now, consulted and built companies on software of my design, and writing code every day, something I do not believe Mr. Huang is doing. Let’s start with a little historical perspective.

Past Promises To Eliminate Programmers
Programming real physical computers in the beginning was a laborious process taking weeks to rewire circuitry with plugboards and switches in the early ENIAC and UNIVAC days.
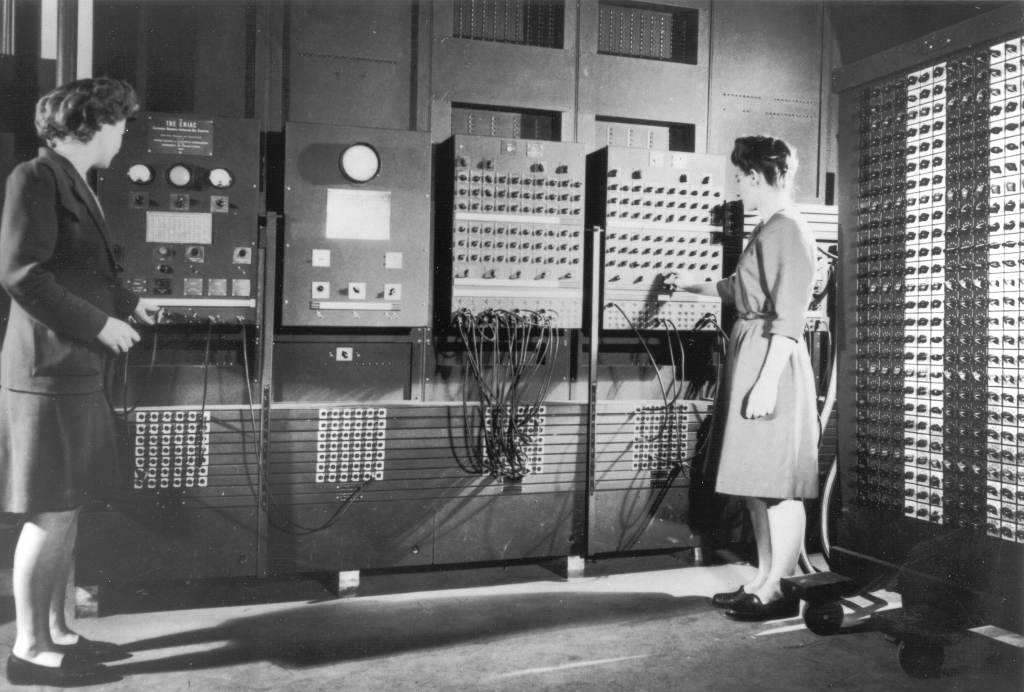
As stored-program machines became more sophisticated and flexible, they could be fed programs in various computer-friendly formats, often via punched cards. These programs were very specific to the architecture of the computer system they were controlling and required specialized knowledge about the computer to make it do what you wanted.

As the utility of computers grew and businesses and government began seeing a need to automate operations, in the 1950’s the U.S. Department of Defense along with industry and academia set up a committee to design a COmmon Business-Oriented Language (COBOL) as a temporary “short-range” solution “good for a year or two” to make the design of business automation software accessible to non-computer-specialists, across a range of industries and fields to promote standards and reusability. The idea was:
Representatives enthusiastically described a language that could work in a wide variety of environments, from banking and insurance to utilities and inventory control. They agreed unanimously that more people should be able to program and that the new language should not be restricted by the limitations of contemporary technology. A majority agreed that the language should make maximal use of English, be capable of change, be machine-independent and be easy to use, even at the expense of power.
The general idea was that now that computers could be instructed in the English language instead of talking to them at their beep-boop level, and programming could be made widely accessible. High-level business requirements could be spelled out in precise business-y language rather than fussing about with operands and memory locations and instruction pointers.
In one sense this certainly was a huge leap forward and did achieve this aim, although to what degree depends on your idea of “widely accessible.” COBOL for business and FORTRAN for science and many many other languages did make programming comparably widely available in contrast to before, although the explosion of personal computers and even more simplified languages like BASIC helped speed things along immensely.
Statistically, the number of people engaged in programming and related computer science fields grew substantially during this period from the 1960s to the year 2000. In the early 1980s, there were approximately 500,000 professional computer programmers in the United States. By the end of the 20th century, this number had grown to approximately 2.3 million programmers due to the tech boom and the widespread adoption of personal computers. The percentage of households owning a computer in the United States increased from 8% in 1984 to 51% in 2000, according to the U.S. Census Bureau. This increase in computer ownership correlates with a greater exposure to programming for the general population.
Every advancement in programming has made the operation of computers more abstract and accessible, which in my opinion is a good thing. It allows programmers to focus on problems closer to providing some value to someone rather than doing undifferentiated mucking about with technical bits and bobs.
As software continues to become more deeply integrated into every aspect of human life, from medicine to agriculture, to entertainment, the military, art, education, transportation, manufacturing, and everything else besides, there has been a need for people to create this software. How many specialists will be needed in the future though?
The U.S. Bureau of Labor Statistics projects the demand for computer programmer jobs in the U.S. to decline 11% from 2022-2032, although notes that 6,700 jobs on average will be created each year due to the need to replace other programmers who may retire (incidentally, COBOL programmers frequently) or exit the market.
So how will AI change this?
Current AI Tooling For Software Development
I’ve been using AI assistants for my daily work, including GitHub’s Copilot for two and a half years, and ChatGPT for the better part of a year. I happily pay for them because they make my life easier and serve valuable functions in helping me to perform some tasks more rapidly. Here’s some of the benefits I get from them:
Finishing what I was going to write anyway.
It’s often the case that I know what code needs to be added to perform some small feature I’m building. Sometimes function by function or line by line, what I’m going to write is mostly obvious based on the surrounding code. There is frequently a natural progression of events, since I’m giving the computer instructions to perform some relatively common task that has been done thousands of times before me by other programmers. Perhaps it’s updating a database record to mark an email as being sent, or updating a customer’s billing status based on the payment of an invoice, or disabling a button while an operation is proceeding. Most of what I’m doing is not groundbreaking never-before-seen stuff, so the next steps in what I’m doing can be anticipated. Giving descriptive names to my files, functions, and variables, and adding descriptive comments is already a great practice for anyone writing maintainable code but also really helps to push Copilot in the right direction. The greatest value here is simply saving me time. Like when you’re writing a reply to an email in Gmail, and it knows how you were going to finish your sentence, so you just tell it to autocomplete what you were going to write anyway, like adding “a great weekend!” to “Have” as a sign-off. Lazy programmers are the most productive programmers and this is a great aid in this pursuit.

Saving me a trip to the documentation.
Most of modern programming is really just gluing other libraries and services together. All of these components you must interface with have their own semantics and vocabulary. If you want to accomplish a task, whether it’s resizing an image or sending a notification to Slack, you typically need to do a quick google search then dig through the documentation of whatever you’re using, which is also often in a multitude of formats and page structures and of highly varying quality. Another time saver is having your AI assistant already aware of how to interface with this particular library or service you’re using, saving you a trip to the documentation.

Writing tests and verifying logic.
It should be mentioned that writing code is actually not all that hard. The real challenge in building software comes in making sure the code you wrote actually does what you expected it to do and doesn’t have bugs. One way we solve this is by writing automated tests that verify the behavior of our code. It doesn’t solve all of our problems but it does add a useful safety net and helps later when you need to modify the code without breaking it. Copilot is useful for automating some of the tedium of this process.
Also sometimes if I have some piece of code with a number of conditions and not very straightforward logic, I will just paste it into ChatGPT and ask me if the logic looks correct. Sometimes it points out potential issues I hadn’t considered or suggests how to rewrite the code to be simpler or more readable.
Finding the appropriate algorithm or formula.
This is less common but sometimes I have some need to perform an operation on data and I don’t know the appropriate algorithm. Suppose I want to get a rough distance between two points on the globe, and I’m more interested in speed rather than accuracy. I don’t know the appropriate formula off the top of my head but I can at least get one suggested to me and it’s ready to convert between the data structures I have available and my desired output format. AIs are great at this.

Problems With “AI” Tooling For Building Software
While the term artificial intelligence is all the buzz right now, there is no intelligence to speak of in any of these commercial tools. They are large language models, which are good at predicting what comes next in a sequence of words, code, etc. I could probably ask one to finish this article for me and maybe it would do a decent job, but I’m not going to.
Fancy autocomplete is powerful and helps with completing something you at least have some idea of how to begin. This sort of tool has fundamental limitations though especially when it comes to software. For one thing, these tools are trained with a finite context window size, meaning they have very small limits in terms of how much information they can work with at any time, can’t really do recursion, and can’t “understand” (using the term very loosely) larger structures of a project.
There are nifty demos of new software being created by AI, which is not such a terribly difficult task. I’ve started hundreds of software projects, there’s no challenge in that. I admit it is very neat to see a napkin drawing of a simple web app turned into code, and this may very well one day in the medium term allow non-technical people to build simple, limited, applications. However, modifying an existing project is a problem that gets drastically harder as the project grows and evolves. Precisely because of the limited context window, an AI agent can’t understand the larger structures that develop in your program. A software program ends up with its own internal representations of data, nomenclatures, interfaces, in essence its own domain-specific programming language for solving its functional requirements. The complexity explodes as each project turns into its own deviation from whatever the LLM has been previously trained on.
Speaking of training, another glaring issue is that computer programmers (I mean, computers that program) only know about what they’ve seen before. Much of that training input is out of date for one thing. Often when I do use a LLM to help me use an API, it will give me commands for an older version of that API which are no longer correct or relevant. New technologies it has particular trouble with, for example giving me all kinds of nonsense about the AWS CDK. But worse than that, anything created after about 2022 or 2023 which be very hard for LLMs to train on because of the “closed loop” problem. This occurs when LLMs begin to learn from their own outputs, a scenario that becomes increasingly likely as they are used to generate more content, including code and documentation. The risk here is twofold: first, there’s the potential for perpetuating inaccuracies. An LLM might generate an incorrect or suboptimal piece of code or explanation, and if this output is ingested back into the training set, the error becomes part of the learning material, potentially leading to a feedback loop of misinformation. Second, this closed loop can lead to a stagnation of knowledge, where the model becomes increasingly confident in its outputs, regardless of their accuracy, because it keeps “seeing” similar examples that it itself generated. This self-reinforcement makes it particularly challenging for LLMs to learn about new libraries, languages, or technologies introduced after their last training cut-off. As a result, the utility of LLMs in programming could be significantly hampered by their inability to stay current with the latest developments, compounded by the risk of circulating and reinforcing their own misconceptions.
If we get rid of most of the programmer specialists as Mr. Huang foresees, then more code generated in the future will be the product of computery-type programmers. They learn by example, but will not always produce the best solution to a given problem, or maybe a great solution but for a slightly different problem. Sure, this can be said about human-style programmers as well, but we’re capable of abstract reasoning and symbolic manipulation in a way that LLMs will never be capable of, meaning we can interrogate the fundamental assumptions being made about how things are done, rather than parroting variations on what’s been seen before and assuming our training data is probably correct because that’s how it was done in the past. Their responses are generated based on patterns in the data they’ve been trained on, not from a process of logical deduction or understanding of a computational problem in the way a human or even a conventional program does. This means that while a LLM can produce code that looks correct, it may not actually function as intended when put into practice, because the model doesn’t truly “understand” the code it’s writing, it’s essentially making educated guesses based on the data it’s seen. While LLMs can replicate patterns and follow templates, they fall short when it comes to genuine innovation or creative problem-solving. The essence of programming often involves thinking outside the box, something that’s inherently difficult for a model that operates by mimicking existing boxes. Even more, programming at its core often involves understanding and manipulating complex systems in ways that are fundamentally abstract and symbolic. This level of abstraction and symbolic manipulation is something that LLMs, as they currently stand, are fundamentally incapable of achieving in a meaningful way.
If these programmer agents do become capable of reasoning and symbolic manipulation, and if they can reason about large amounts of data, then we will be truly living in a different world. People with money and brains are actively working on these projects and there are financial and reputational incentives now motivating this work in a major way, so I absolutely expect advancements one day. How far that day is off, I dare not speculate on.
The Users Of Computer-Aided Programming Tools
One of the coolest features and biggest footguns about programming is that you can give a computer instructions that it will follow perfectly and without fail exactly as you specify them. I think it’s amazingly neat to have a robot that will do whatever you ask it, never get tired, never need oiling, can be shared and tinkered with, worked on in maximum comfort from anywhere. That’s partly what I love about programming, I enjoy bossing a machine around and imposing my will on it, and it almost never complains. But the problem anyone learns almost immediately when they start writing code is that computers will do exactly what you tell them to do without any idea of what you want them to do. When people communicate with each other there is a huge background of shared context, not to mention gestures, facial expressions, tones, and other verbal and non-verbal channels to help get the message across more accurately. ChatGPT does not seem to get the hint even when I get so frustrated I start swearing at it. Colleagues that work together on a shared task in the context of a larger organization have a shared understanding of purpose, risks, culture, legal environment, etc. that they are operating inside of. Even with all of this, human programmers still err constantly in translating things like business requirements into computer programs. A LLM with none of this out-of-band information will have a much harder time and make many more assumptions than a colleague performing the same task. The LLM may however be better suited for narrowly-defined, well-scoped tasks which are not doing anything new. I hope and believe LLMs will reduce drudgery, boilerplate, and wheel reinventing. But I wouldn’t count on them excelling at building anything new, partly because of how non-specialists will try to communicate with them.
In most professions a sort of jargon develops. Practitioners learn the special meanings of words in their fields, for example in medicine or law or baseball. In the world of software we love applying 10-dollar words like “polymorphism” or “atomicity” to justify our inflated paychecks, but there is another benefit to this jargon, which is it can help us describe common concepts and ideas more precisely. If you say to a coder to “add up all the stuff we sold yesterday”, you may or may not get the answer to the question you think you’re asking. If you say “sum the invoice totals for all transactions from 2024-04-01 at 00:00 UTC up to 2024-04-02 00:00 UTC for accounts in the USA” you are a lot more likely to get the answer you want and be more confident that you got a reliable number. Less precise instructions leave huge mists of ambiguity which programming computers will happily fill in with assumptions. This is where much of the value of specialists comes in, namely the facility in some dialect which enables a greater precision of expression and intent than the verbiage someone in the Sales or HR department will reliably deploy. In plenty of situations, say if you’re building a social media network for parakeets, maybe it’s okay. But when real money is on the line, or health and safety, or decisions with liability ramifications are introduced, I predict companies will still want to keep one or two specialists around to make sure they aren’t now playing a game of telephone with a coworker talking to a LLM on the other end and who knows what logic emerging out of the other end.
Introducing AI agents into your software development flow may create more problems than it solves, at least with what is likely to be around in the near term. On the terrific JS Party podcast they recently took a look at “Devin”, a brand new tool which claims the ability to go off by itself and complete tickets like a software engineer in your team might do. It received heaps of breathless press. But until these agents are actually reliable, other engineers still will have to debug the work done by the agent, which could end up wasting more time than is saved.
But the number they published, I think, was 13.86% of issues unresolved. So that’s about one in seven. So you pointed out a list of issues, and it can independently go and solve one in seven. And first off, to me, I’m like “That is not an independent software developer.” And furthermore, I find myself asking “If its success rate is one in seven, how do you know which one?” Are the other six those “It just got stuck”? Or has it submitted something broken? Because if it sets up something broken, that doesn’t actually solve the issue, not only do you have it only actually solving one in seven, but you’ve added load, because you have to go and debug and figure out which things are broken. So I think the marketing stance there is little over the top relative to what’s being delivered.
The software YouTuber Internet of Bugs went on to accuse the creators of Devin of misleading the public about its capabilities in a detailed takedown.
And in practice, a lot of code a LLM gives me is just wrong. A couple weeks ago I think I ended up trying to write a script for around four hours with ChatGPT when probably should have just looked up the relevant documentation myself. It kept going in circles and giving me code that just didn’t do what it said it did, even with me feeding it back output and error messages.
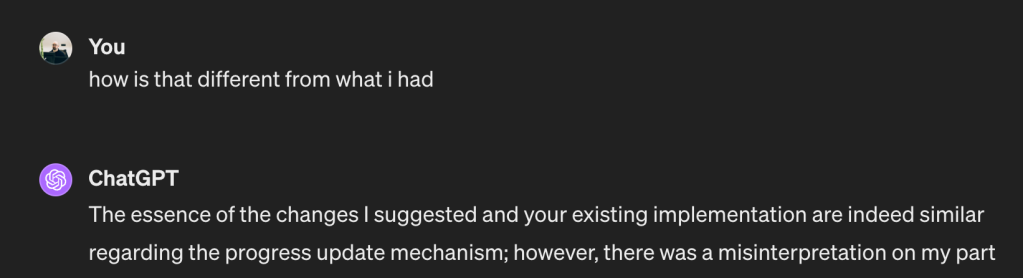
What’s The Future?
While there are many reasons for skepticism about AI-assisted programming, I am guardedly optimistic. I do get value out of the tools I use today which I believe will improve rapidly. I know there are very smart people out there working on them, assisted by computers making the tools even smarter, perhaps not unlike how computers started to be used to design better processors in a powerful feedback loop. I have limited expectations for LLMs but they are certainly not the only tool in the AI toolbox and there are vast amounts of money flowing into research and development, some of which will undoubtedly produce results. Computers will be able to drop some of the arbitrary strictness that made programming so tedious in the past and present (perhaps a smarter IDE will not bother you ever again about forgetting a ;
or about an obviously mistyped variable name). I have high hopes for better static analysis tools or automated code reviews. Long-term I do believe how most people interact with computers will fundamentally change. AI agents will be trusted with more and more real-world tasks in the way that more and more vital societal functions have been allowed to be conducted over the internet. But someone will still have to create those agents and the systems they interact with and it won’t be only AI agents talking to themselves. At least I sure hope not.
So I’m not too concerned for my job security just yet. Software continues to eat the world and I’m not convinced the current state of the art can do the job of programmers without specialist intermediaries. As in the past, new development tools and paradigms will make programmers more productive and able to spend more time focusing on the problems that need solving rather than on mundane tasks. Maybe we will be able to let computers automate their automation of our lives but it will be on the less-mission-critical margins for quite some time.